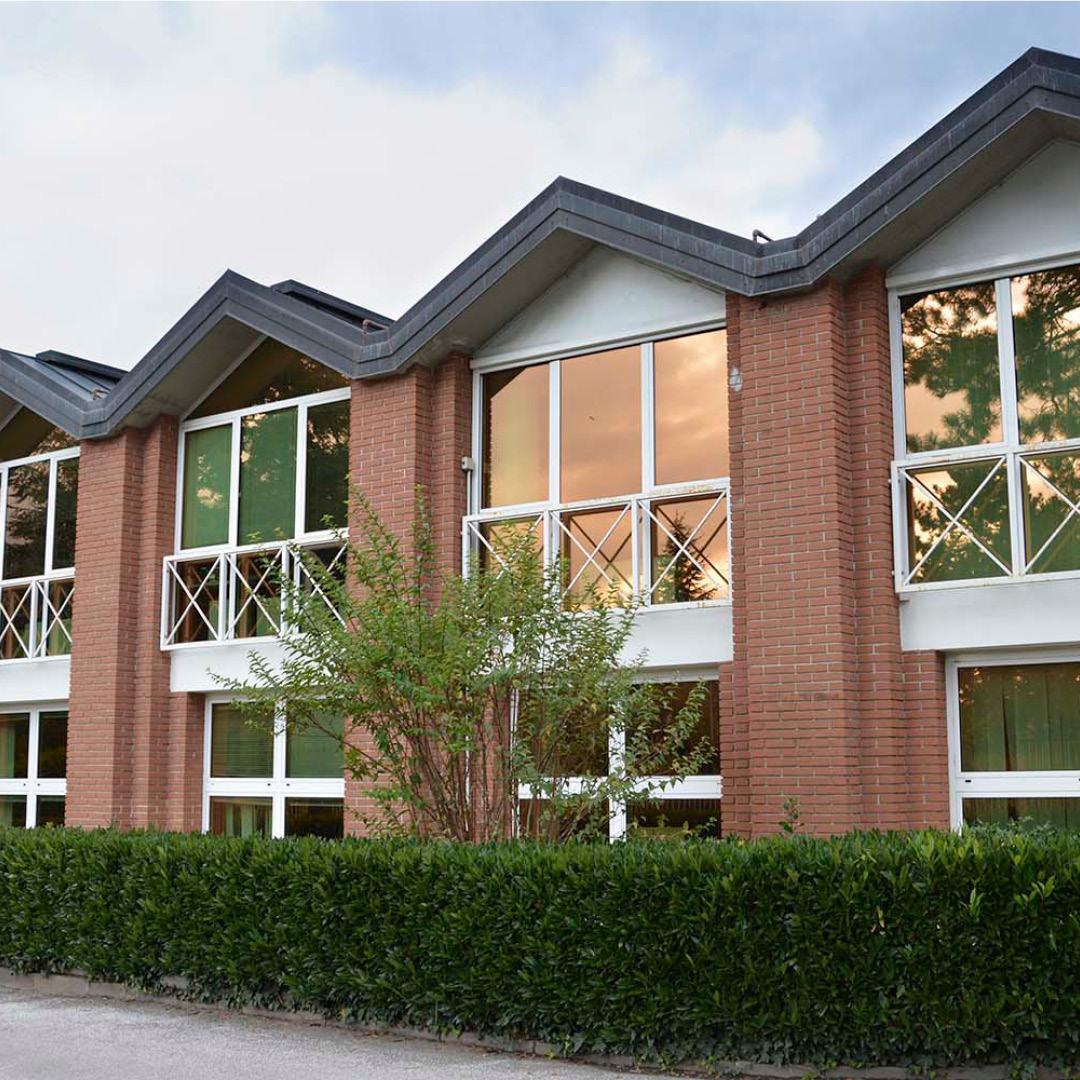
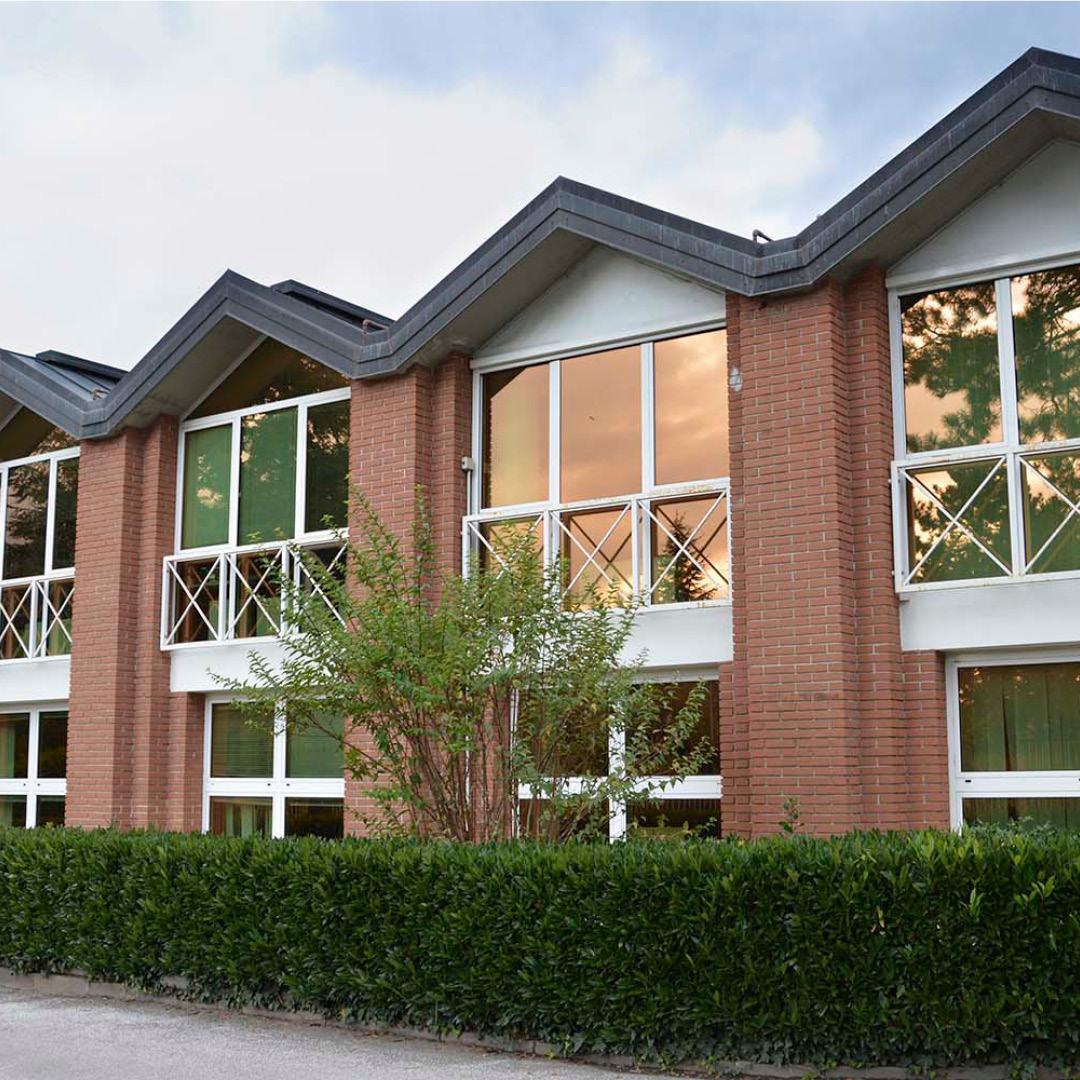
Abstract:
Given a stochastic process, a mimicking theorem proves the existence of a stochastic differential equation whose probability distribution is equal to the probability distribution of the stochastic process. Previous results rely on martingale
techniques, but in this talk I will describe a class of stochastic processes which are not semimartingales but can be mimicked. The most well known examples include stochastic processes driven by an additive fractional Brownian
motion, but we include necessary conditions which allow for other Gaussian noises.
One application of mimicking theorems is in the use of a BBGKY hierarchy to calculate sharp rates of convergence for the relative entropy between the marginal dynamics of interacting particle systems and their mean-field limits. The rate
of convergence achieved is O( (k/n)^2), which exceeds more general results and is optimal for linear SDEs.
This is joint work with Kavita Ramanan and Kevin Hu.