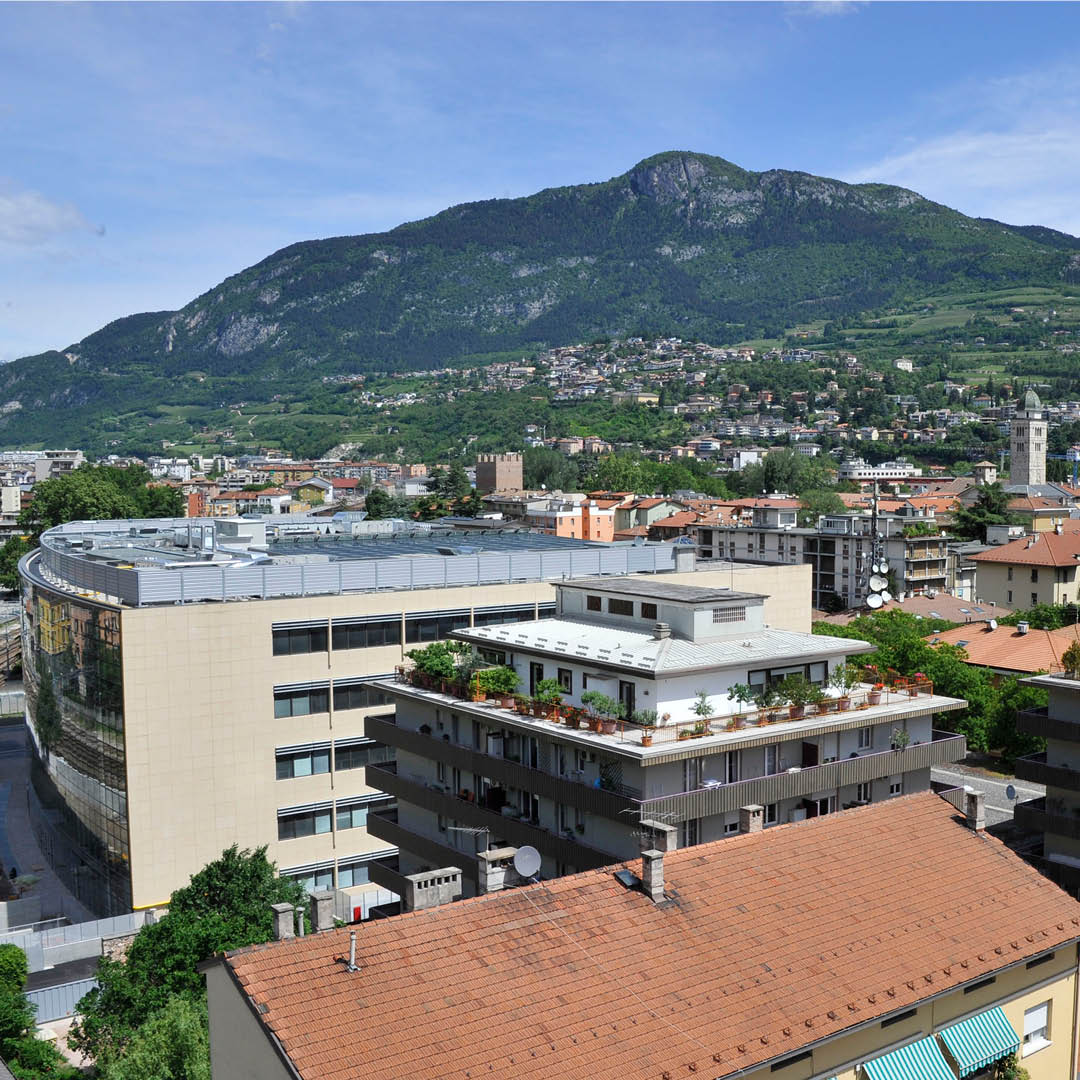
Integration of Machine Learning with Dempster-Shafer Theory for User Preferences Modeling
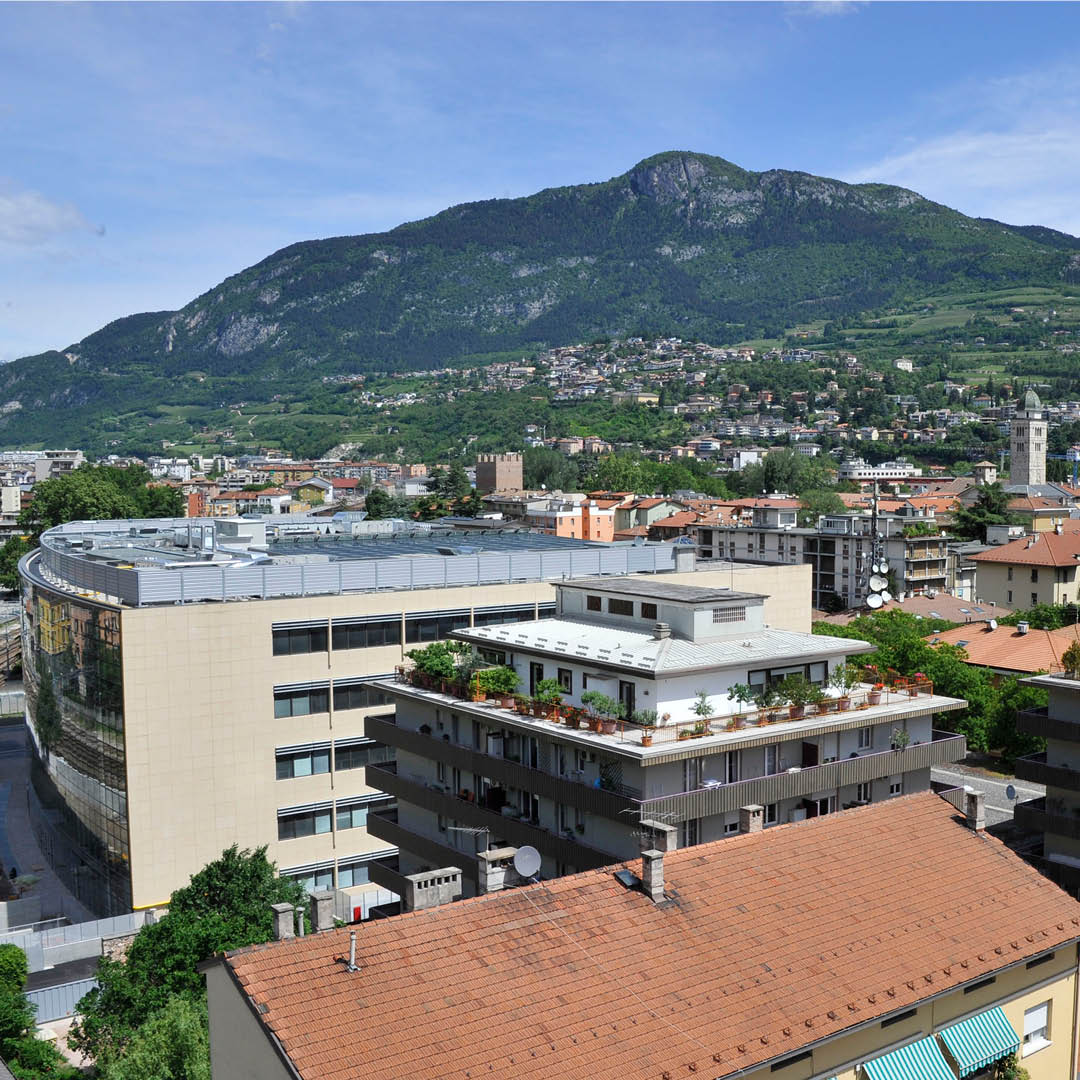
In the first part of the talk, a brief introduction to Decision Intelligence Lab at Japan Advanced Institute of Science and Technology and two research projects on Kansei engineering and explainable machine learning under investigation at the lab will be given.
In the second part, the problem of user profile learning will be introduced and an integrated approach for user profile learning will be discussed.
User profiles that represent users’ preferences and interests play an important role in many applications of personalization. With the rapid growth of multiple social platforms, there is a critical need for efficient solutions to learn user profiles from the information shared by users on social platforms so as to improve the quality of personalized services in online environments. Developing an efficient solution to the problem of user profile learning is significantly challenging due to difficulty in handling data from multiple sources, in different formats and often associated with uncertainty. In this talk, an integrated framework that combines advanced Machine Learning techniques with Dempster-Shafer theory of evidence (DST), a mathematical framework for representation and combination of uncertain data and information, for user profiling and recommendation, will be introduced.
Two instances of the proposed framework for user profile learning and multi-criteria collaborative filtering will be demonstrated with experimental results and analysis that show the effectiveness and practicality of the developed methods.
Finally, the potential of integrating advanced ML techniques with DST for user preferences modeling is highlighted.