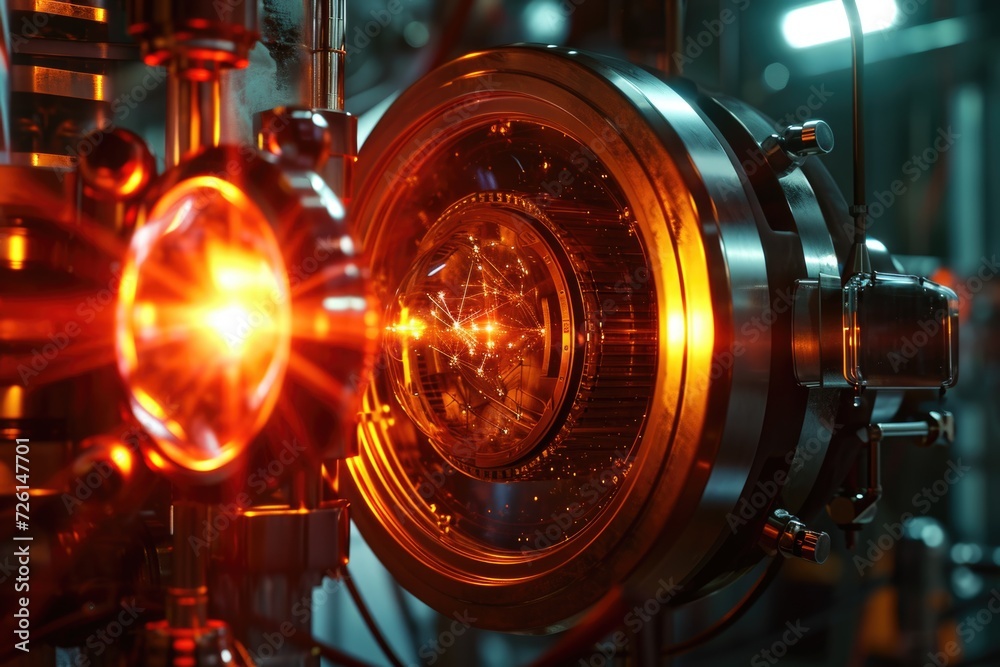
Trained quantum neural networks are Gaussian processes
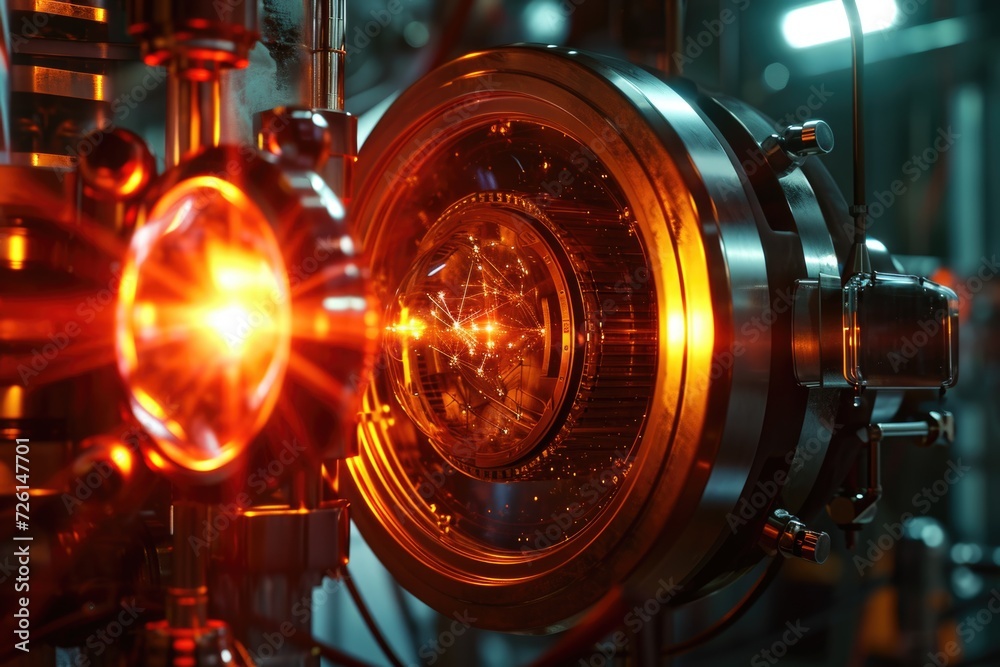
- ricerca
Abstract
Trained quantum neural networks are Gaussian processes
Quantum neural networks represent the quantum analog of deep neural networks, leveraging the unique properties of quantum mechanics to potentially enhance machine-learning algorithms. Despite their promise, quantum neural networks currently lack a solid mathematical foundation. This work aims to establish such foundation. We investigate quantum neural networks for supervised learning, constructed with parametric one-qubit gates and fixed two-qubit gates, where the output function is the expectation value of the sum of single-qubit observables across all qubits. First, we demonstrate that the probability distribution of the function generated by untrained quantum neural networks with randomly initialized parameters converges in distribution to a Gaussian process in the limit of infinite width, provided each measured qubit is correlated with only a few other qubits. Then, we analytically characterize the gradient-descent training dynamics of the network in the limit of infinite width. We prove that the loss function decays exponentially in the training time, and therefore that the trained network can perfectly fit the training set. Moreover, we prove that during the whole training, the probability distribution of the generated function still converges in distribution to a Gaussian process. The proof of such result relies on proving that training occurs in the lazy regime, i.e., that the maximum variation of each parameter vanishes in the limit of infinite width. Finally, we address the statistical noise in measurements at the output of the network, proving that number of measurements growing polynomially with the number of qubits is sufficient to ensure the convergence to a Gaussian process, and therefore that the network can be trained in polynomial time.