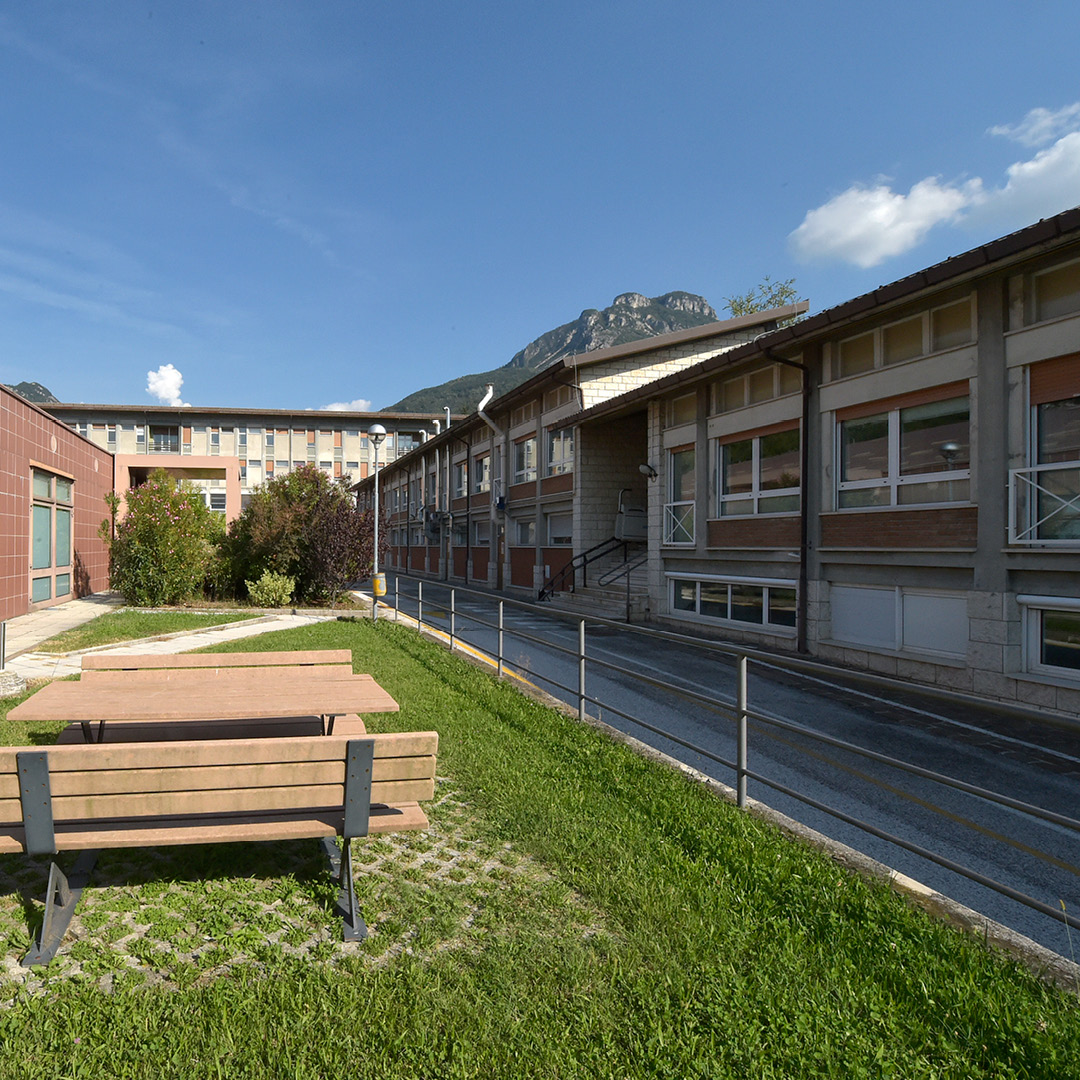
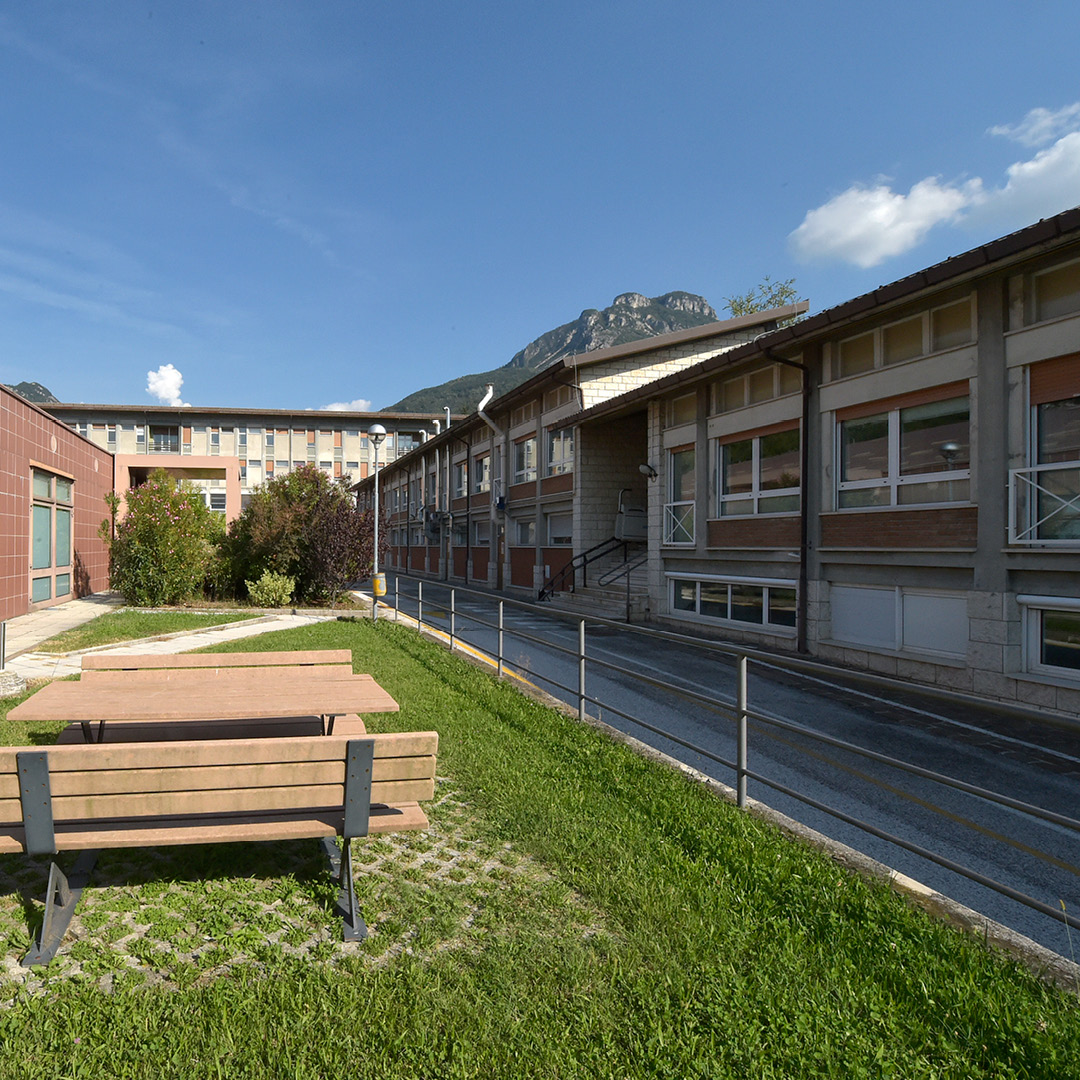
This content is only available in Italian.
Abstract
Transformers are central to state-of-the-art performance in large language models such as ChatGPT. In this talk, we model the evolution of tokens within deep Transformer architectures as a continuous-time, mean-field interacting particle system on the unit sphere, based on the framework from [GLPR23]. We analyze the associated mean-field PDE, interpreted as a Wasserstein gradient flow, to investigate the emergence of meta-stable phases and clustering phenomena, relevant for next-token prediction. Using perturbative analysis around uniform initialization, we show that for a large number of tokens, the system remains near a structured meta-stable manifold. This structure, characterized by rescaled Gegenbauer polynomials, is explicitly linked to the inverse temperature parameter, providing insights into mean-field dynamics and emergent behaviors in Transformer models.
This is joint work with Giuseppe Bruno and Federico Pasqualotto. [GLPR23] Geshkovski, Letrouit, Polyanskiy, and Rigollet. A mathematical perspective on transformers. arXiv:2312.10794, 2023
Email: